JOB OPENING: POSTDOCTORAL POSITION
2023/02/03 | projects
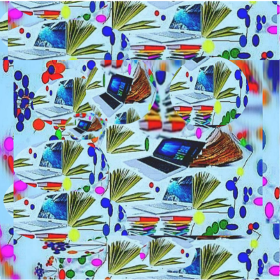
Postdoctoral position in Network Science - Machine Learning
The Mapping Complexity Lab of Prof. M. Ángeles Serrano and Prof. M. Boguñá is opening a call to hire a postdoctoral researcher in the Department of Condensed Matter Physics at the University of Barcelona.
Requirements:
A PhD in physics, computer science, computer/electronic engineering, mathematics, or other related disciplines.
Interest in interdisciplinary research, curiosity about AI and networks, high motivation to learn, an open-minded and collaborative spirit.
Excellent software development skills.
Excellent communication skills, and proficient in the English language, both written and spoken.
Offer:
The successful applicant will work with Prof. M. Ángeles Serrano and Prof. Marián Boguñá at the interface between Network Science and Machine Learning. The goal is to merge the best of the two worlds to produce a new generation of models and methods for the classification and prediction of complex networks. We offer a 2-year position (1+1) with a competitive salary.
Application process:
Interested applicants are requested to submit a Curriculum Vitae including relevant publications and the name and contact details of 2 referees.
We promote diversity and equal opportunities, minorities in science are encouraged to apply.
Queries about this position should be sent to marian.serrano@ub.edu or marian.boguna@ub.edu